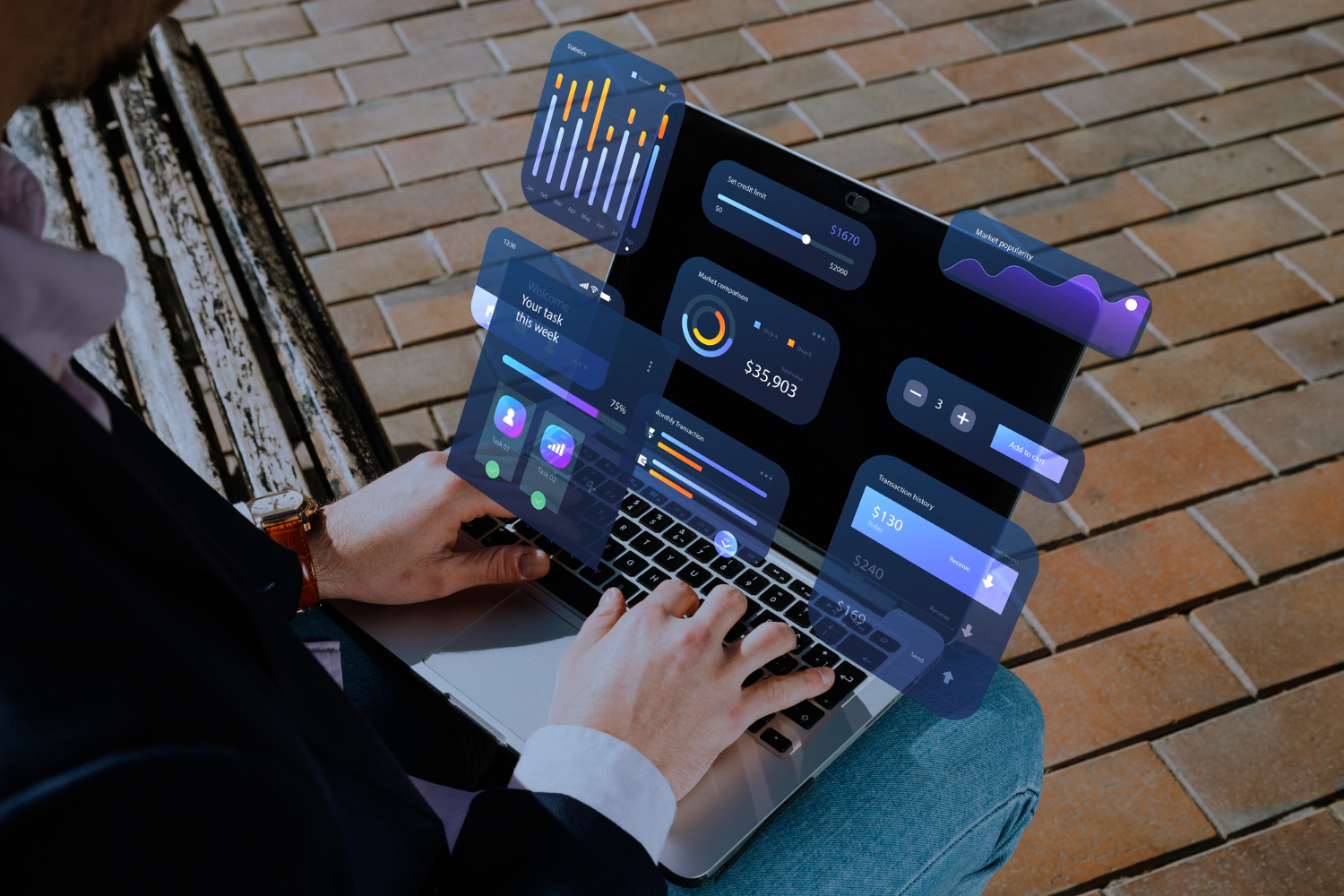
Data Science: Transforming Raw Data into Business Intelligence
In today’s data-driven economy, organizations generate vast amounts of raw data daily—from customer transactions and web behavior to sensor readings and social media feedback. Yet, this raw data holds no intrinsic value unless it is transformed into meaningful insights. Turning data science into business intelligence is what empowers companies to make informed decisions, optimize operations, and gain a competitive edge.
Businesses across sectors are now integrating data science practices to extract strategic insights from complex datasets. This transformation from raw data to actionable intelligence marks a pivotal shift in how modern enterprises operate.
Key Themes Explored
- The role of data science in decoding raw data
- The bridge between data science and business intelligence
- Industry use cases that highlight real-world impact
- Practical steps businesses can take to implement data science
- Key benefits and ROI of using data science tools and techniques
Understanding the Power of Data Science in Business Intelligence
Data science into business intelligence refers to the application of advanced analytical techniques—such as machine learning, predictive modeling, and statistical analysis—to transform large, unstructured datasets into digestible, decision-making tools.
Where traditional business intelligence answers what happened, data science dives deeper into why it happened and what will happen next. This evolution is critical for proactive, not just reactive, business strategies.
Real-World Applications of Data Science into Business Intelligence
- Retail: Companies like Amazon and Walmart use data science to analyze buying behavior and optimize inventory and supply chains. Through recommendation engines, they boost sales and personalize customer experiences.
- Healthcare: Hospitals utilize predictive analytics to anticipate patient admissions, optimize staff allocation, and improve treatment outcomes based on patient history.
- Finance: Banks deploy machine learning to detect fraudulent activities in real time and assess credit risk, enhancing both security and customer trust.
- Manufacturing: Data from IoT sensors is processed to predict equipment failures, improving maintenance cycles and reducing downtime.
Key Statistics and Industry Insights
- According to McKinsey, companies that integrate data science practices into decision-making see profit gains of up to 6% over competitors.
- Gartner reports that by 2026, 75% of businesses will have shifted from traditional BI to automated, insight-driven decision-making enabled by data science.
- A Deloitte survey shows 67% of high-performing companies regard data science as a key driver of innovation and competitive advantage.
Core Components of Data Science into Business Intelligence
- Data Collection: Gathering structured and unstructured data from diverse sources
- Data Cleaning and Preparation: Removing inconsistencies and formatting for analysis
- Exploratory Data Analysis: Identifying trends, anomalies, and patterns
- Modeling and Prediction: Applying algorithms to forecast outcomes
- Visualization and Reporting: Converting insights into dashboards and reports
- Integration with BI Tools: Feeding analytical results into platforms like Power BI, Tableau, or Looker
Steps to Integrate Data Science into Your Business Intelligence Workflow
- Define Clear Business Objectives
Align your data science initiatives with specific goals—like increasing sales, improving customer retention, or reducing costs. - Invest in Data Infrastructure
Ensure secure data storage, seamless data integration pipelines, and scalable cloud platforms. - Build a Cross-Functional Team
Combine the expertise of data scientists, domain experts, and business analysts to interpret data meaningfully. - Select the Right Tools
Utilize tools such as Python, R, SQL, Power BI, and TensorFlow to analyze and visualize your data effectively. - Develop Scalable Models
Start with pilot models and iterate based on feedback and performance. Deploy models into production when refined. - Embed Insights into Decision Processes
Ensure that insights derived from data science are easily accessible to decision-makers through dashboards and alerts. - Train Staff and Encourage Data Literacy
Promote a data-first culture by educating teams on reading dashboards, understanding models, and making evidence-based decisions.
Benefits of Applying Data Science into Business Intelligence
- Better Forecasting and Planning: Predict future trends with greater accuracy
- Enhanced Customer Experience: Personalize interactions and offerings based on behavioral insights
- Operational Efficiency: Identify inefficiencies and streamline processes
- Risk Management: Detect fraud and monitor compliance in real time
- Strategic Advantage: Stay ahead of competitors by acting on early insights
Data alone is not a competitive advantage—but turning that data into actionable intelligence is. By implementing data science into business intelligence, organizations unlock the full potential of their data assets. This fusion leads to smarter decisions, improved operational outcomes, and a culture of continuous innovation.
For businesses aiming to thrive in an increasingly data-centric world, now is the time to integrate data science not as a luxury—but as a core strategic capability.